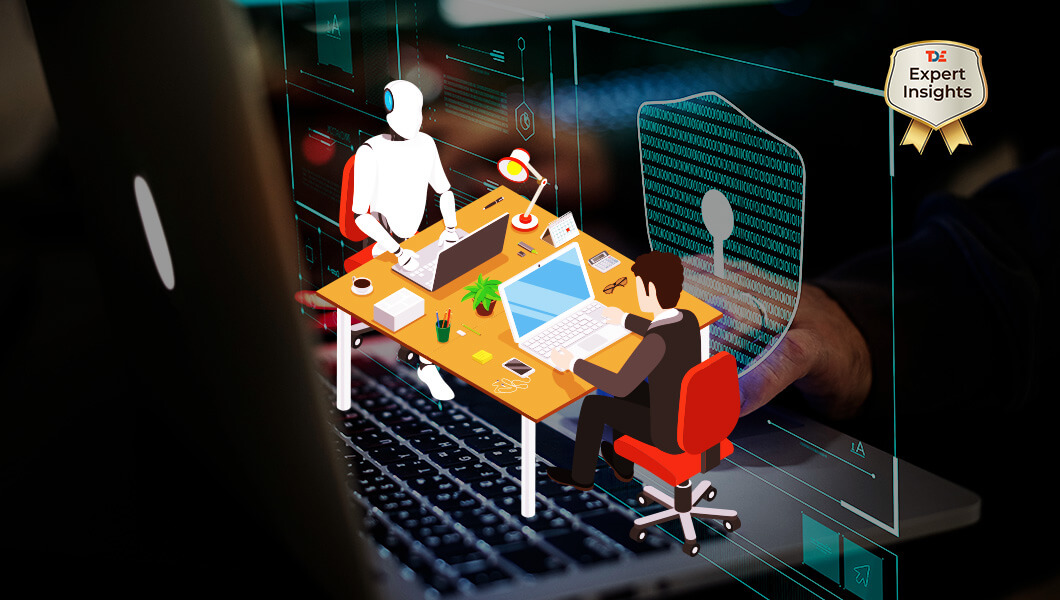
AI and machine learning have transformed cyber security strategies and help in managing skills and resource gaps and minimize human error for data breaches. Nevertheless, E J Whaley, Solutions Engineer at GreatHorn advises against over-reliance on technology and suggests IT and security professionals audit automation to ensure it doesn’t hamper efficiency of business operations
As computing at work and at home has become more widespread and the accompanying risks have grown, a number of cure-alls or silver bullets to address these risks have entered the lexicon. Most recently, we’ve seen endpoint detection and response take this role; prior to that, it was access and device management, and before that antivirus… how many times can organizations affix “next-generation” to their platforms, or redefine what their product does by calling it something different? Seemingly product development and improvement is no longer implicit.
These are only a handful of tools that have been touted as cybersecurity “silver bullets” – the answer to how we finally get security under control. But, we still run into a number of the same problems we were running into before these technologies were introduced and, frankly, that is not surprising: attackers adapt, landscapes shift, as new technologies not accounted for by existing security tooling are adopted, and humans are fallible. While each of the aforementioned technologies, as well as countless others for that matter, have contributed significantly to addressing a hole in our security arsenal, none of these have lived up to their lofty expectations of being a panacea to our security woes. We’ve seen this all too often, despite learning over and over again that security is complex and there simply is no single fix.
As an industry – and really, as humans – we have a tendency to look at valuable new technology and try to apply it indiscriminately to every problem we have. It’s like the old adage – if you have a hammer, suddenly everything starts to look like a nail. As a result, we end up in a cycle where overhype leads to outsized expectations which then, more often than not, leads to some level of disappointment. Technologies, which were purportedly the end of a problem, only created new ones, while not always completely addressing the old ones.
Enter the latest poster child for this cycle: Artificial Intelligence (AI) and, more specifically, machine learning (ML). To avoid the backlash that has plagued so many security tools before it, IT leaders should have a realistic expectation of what ML can – and cannot – do to help their cybersecurity strategy.
Machine Learning in Security Needs Supervision
The first question is – what is the machine supposed to learn? The value of machine learning to the cybersecurity realm is in the ability to more accurately and precisely identify, understand, and protect areas of vulnerability. But, as with most AI initiatives, human oversight is still vital. Imagine that you work in an office building where the smoke detector goes off regularly, sometimes multiple times a day. Each time the alarm goes off, the people inside are not given any context, so each time it sounds there is uncertainty as to what constitutes appropriate action. While it doesn’t make sense to evacuate every time someone burns popcorn in the company microwave, inaction in the face of a real threat could have dire consequences. This example showcases the most important question security leaders need to consider when deciding how and when to implement automation: which decisions can software reasonably make better than a human, and when is human intervention not only desired, but required for a positive result?
Enterprises can get themselves into trouble utilizing automation within tooling when there is a complete or near complete reliance on the technology to be deterministic. Organizations often say they aren’t looking for these silver bullets on projects, but many still rely on a set of point solutions with limited-to-no overlap, believing fervently that each tool will accomplish its job and the organization will be secure. Sometimes this is out of necessity – lack of budget for additional tools, lack of employees to run them, or lack of support for security initiatives internally – but, in others, it is an overreliance and misplaced trust in the claims of the technology.
Certainly, machine learning coupled with automation can drive information security costs down and enable organizations to redeploy expensive staff to other critical areas. However, what is often misunderstood is how much these technologies can do with exactitude. Many teams want the technology or software to provide definitive answers for every scenario, but that’s simply not possible. We can create thresholds, and we can program the software to take definitive action when those thresholds are met or exceeded. However, what do we do with those exceptions that meet the policy threshold but are in fact innocuous? With teams still highly sensitive to false-positives (and rightfully so), this creates a no-win situation, where businesses want the software to make decisions, but label it a failure when the software gets it wrong.
Positioning for Success in Security Needs
When it comes to identifying patterns, anomalies or breaks in processes across a wide array of data, machines have the upper hand. Identifying such breaks and adapting algorithms to accommodate new patterns of behavior is where machine learning can be exceptionally useful. IT and security leaders that oversee hundreds-to-thousands of users and hundreds-to-thousands of machines simply will not be able to perform these types of analyses in a scalable fashion. The ability for software to quickly aggregate data, perform correlation across previously seen or ongoing processes, and convert that analysis into actionable information far outpaces what humans can process.
But, organizations should consider how reliant on that analysis they should, or even want to be. Is a string of previously unseen code malicious, or are the developers working on something new? If it’s the latter, and the software responsible for detecting anomalous behaviors prevents the new code from executing, you slow the team and development. Indiscriminately automating action on known bad code attempting to execute on developers’ machines creates both business process and security gaps. In many cases, security vulnerabilities are a result of business process gaps, or they exist because of a conscious trade-off between strong security and business operation efficiency. That said, humans have a variety of context for the information they see and can make better judgments in those situations: this set of machines belongs to the developers, and the organization has put in place a number of contingencies, both automated and manual, to ensure security without introducing crippling inefficiencies.
Take, as another example, corporate email security. Every week, enterprises receive an average of 3,680 emails displaying characteristics indicative of a threat. This type of volume creates an overwhelming amount of administrative work for most IT and / or security teams. Through the use of machine learning, security leaders can help their teams more efficiently manage the large number of potential vulnerabilities they face by programmatically identifying and addressing threats based on preset policies that take into account what the system is able to glean and then set actions appropriately.
Automation is able to increase threat detection accuracy by leveraging machine learning to determine whether an individual message is an attempt to deceive or phish an organization’s employees. This is accomplished by analyzing metadata such as email authentication and return path, as well as relationship strength between the sender and recipient. Is this Aunt Sue sending an email with an off-color joke with a questionable URL, or is it a phishing attempt? These data points increase the visibility of phishing threats, reduce the time it takes for security teams to respond to threats, and can detect patterns that a human might otherwise miss.
Yet humans should be involved in configuring policies to correspond with an organization’s risk tolerance, creating new ones based on an organization’s unique business processes, and tuning the system when and where efficacy is low and taking cues from where efficacy is high. Additionally, rather than quarantining each and every questionable message (or constantly tuning thresholds to increase or decrease the number of messages being quarantined) and requiring administrator intervention, organizations can take risk-appropriate (automated or manual, not always manual review) action based upon the level of threat demonstrated in the email.
Ultimately, the key to unlocking the potential of machine learning is for security leaders to understand their existing team, tooling, and processes. Without understanding where the IT team has strengths and weaknesses in their processes and team, their efforts may all be for naught. A strategic rollout approach after a thorough audit of systems and policies will highlight highly manual tasks, where machine learning can provide significant value to a business and where the organization will benefit from human intervention.